AIM Science
We build bridges between medicine and technology
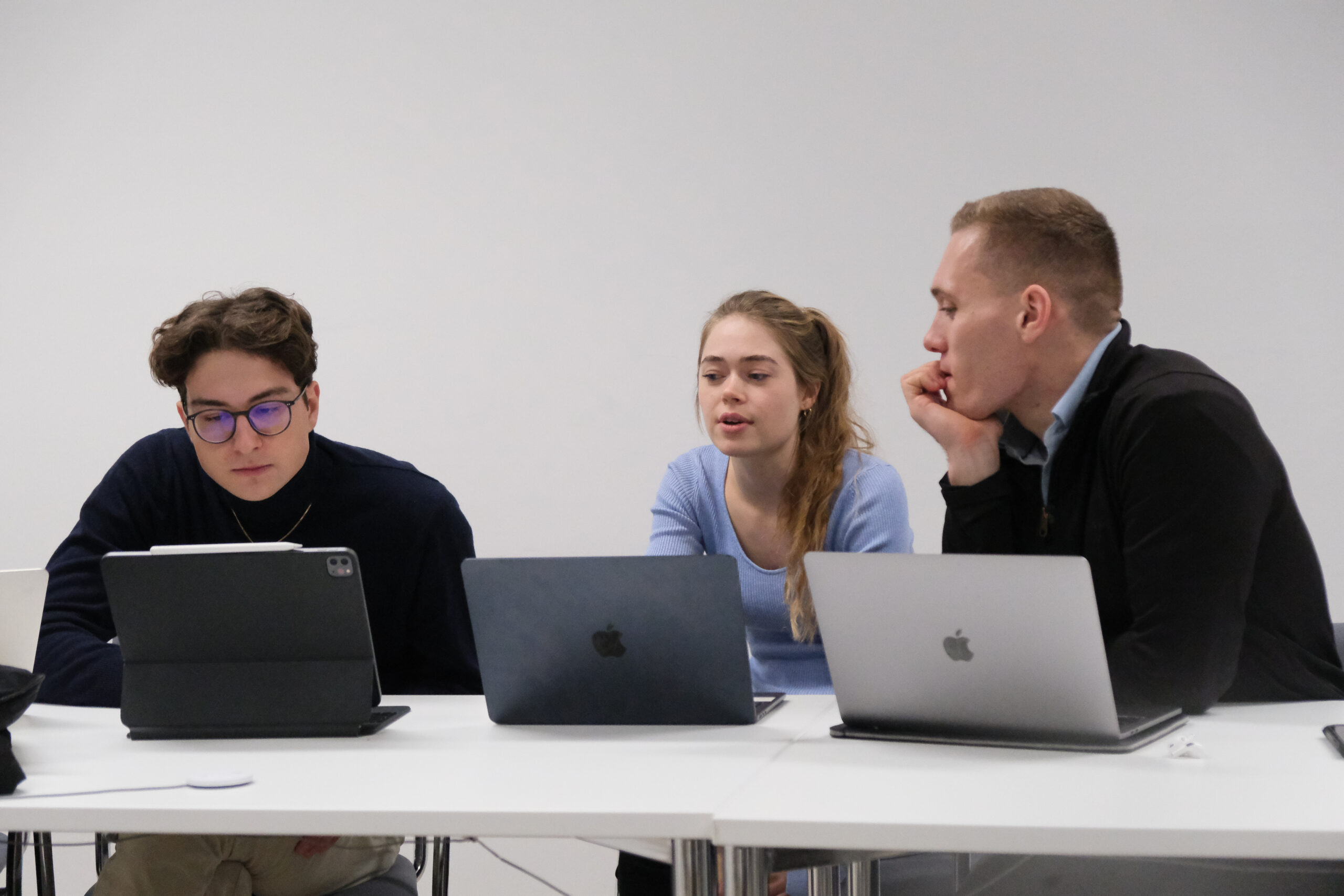
Our publications
Demographic Inaccuracies and Biases in the Depiction of Patients by Artificial Intelligence Text-to-Image Generators
The wide usage of artificial intelligence (AI) text-to-image generators raises concerns about the role of AI in amplifying misconceptions in healthcare. This study therefore evaluated the demographic accuracy and potential biases in the depiction of patients by two commonly used text-to-image generators. A total of 4,580 images of patients with 29 different diseases was generated using the Bing Image Generator and Meta Imagine. Eight independent raters determined the sex, age, weight group, and race and ethnicity of the patients depicted. Comparison to the real-world epidemiology showed that the generated images failed to depict demographical characteristics such as sex, age, and race and ethnicity accurately. In addition, we observed an over-representation of White as well as normal weight individuals. Inaccuracies and biases may stem from non-representative and non-specific training data as well as insufficient or misdirected bias mitigation strategies. In consequence, new strategies to counteract such inaccuracies and biases are needed.
https://www.researchsquare.com/article/rs-4523331/v1
ChatGPT Passes German State Examination in Medicine With Picture Questions Omitted
Full medical registration in Germany requires passing three medical state examinations. The first state exam (M1) regarding pre-clinical subjects consists of a written exam and a viva voce exam. The second state exam (M2) is a written test and comprises the medical specialties. The third state exam (M3)is an oral/viva voce practical exam. The written test questions are published by the German Institute for Medical and Pharmaceutical Examination Questions (IMPP).Both written tests consist of 320 single choice questions with five possible answers each.
Recently, artificial intelligence (AI) in the form of ChatGPT passed examinations of the US Medical License exam (USMLE). ChatGPT is a large language model (LLM), which is based on the transformer network architecture “general pre-trained transformer” (GPT) with more than 170 billion parameters. It recognizes speech patterns and responds in a context appropriate way to user queries/questions.
We investigated whether Chat GPT could pass the first and second state examinations in Germany and thus is able to answer complex medical questions in the German language.
Künstliche Intelligenz in der Neurologie: Anwendungen, Algorithmen, Anforderungen
Künstliche Intelligenz (KI) kann große Datenmengen verarbeiten und interpretieren und birgt daher großes Potenzial für die Neurologie. Viele neurologische Erkrankungen erfordern umfangreiche multimodale Diagnostik und personalisierte Therapiekonzepte, die durch KI automatisiert bzw. optimiert werden können. Hinter der KI verbergen sich komplexe Algorithmen. Ziel dieser Arbeit ist es, einen Überblick über Grundbegriffe, Algorithmen und Anwendungen der KI in der Neurologie zu vermitteln. Es existiert eine Vielzahl an KI-Modellen. Besonders leistungsstark sind neuronale Netze und Transformer. Neuronale Netze können durch die Verarbeitung statischer Eingabedaten, z. B. von radiologischen Bildern, Verdachtsdiagnosen oder Prognoseeinschätzungen abgeben. Ihre Funktionsweise basiert auf einer großen Anzahl kleiner Recheneinheiten, den Neuronen, die zu Netzen angeordnet sind. Transformer hingegen können sequenzielle Daten wie Text verarbeiten und somit, z. B. basierend auf Anamnesegesprächen, Verdachtsdiagnosen ausgeben oder Befundberichte formulieren. Sie berechnen hierzu die Beziehung der einzelnen Textsegmente zueinander, sodass diese bei der Verarbeitung berücksichtigt werden können. Ein grundlegendes Verständnis der Anwendungen und Funktionsweisen der KI, aber auch der Herausforderungen und Limitationen ist für den erfolgreichen Einsatz in der neurologischen Forschung und Praxis entscheidend.
https://www.thieme-connect.de/products/ejournals/abstract/10.1055/a-2050-0768